Call us toll-free: 800-878-7828 — Monday - Friday — 8AM - 5PM EST
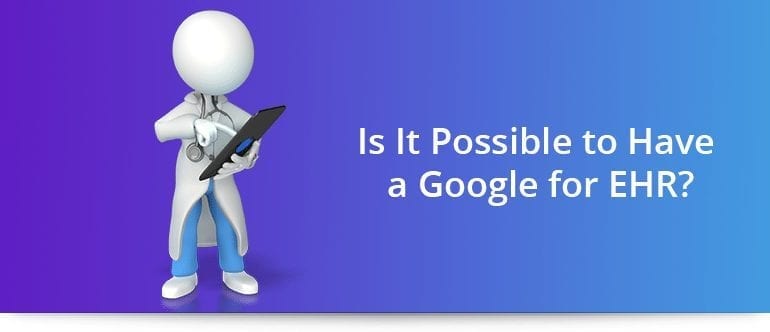
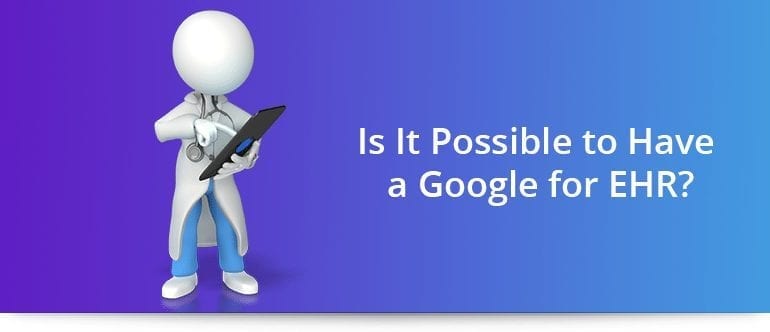
By Jasmine Morgan for Technology Networks
Medical records are some of the richest and most under-utilized data sources. Imagine if you could search medical records in the same way you search Google. This would require that all the information was properly categorized and tagged. Such a tool would offer all members of the medical staff the opportunity to find the information they need and speed up healthcare whilst diminishing errors.
The current problem is that most hospitals and clinics have different, unconnected, data storage systems. The EHR is mostly maintained manually and can suffer from delayed updates. Secondly, notes by doctors and nurses are usually written on paper. These are translated to digital storage using disease codes. Unfortunately, these codes are not correlated and don’t tell the whole story.
Why Is Medical Coding Not Enough?
Medical codes were introduced from an accountant’s perspective. Their role is to help insurance companies reimburse hospitals for their efforts to cure the patients. Coding is a way to create a common language between the physician and the financial department, as well as between different medical facilities.
Although codes recorded in the EHR are a good starting point for disease analysis, these need to be correlated with each other and with other data from the physician’s observation sheet. Currently, there are numerous coding schemes in place, but there is no centralized search tool.
Also, it’s worth mentioning at this point that any human error in medical coding can create both material loses and a misunderstanding of the disease.
A Google for Healthcare
The goal would be to create a tool for the medical system that is as user-friendly as Google Search but has access to private medical data. If designed, such an engine would offer insights on both individual cases and general outcomes for a combination of factors by aggregating data from large data lakes.
Such a tool would be most useful if it could be understood and used by any of the stakeholders in the medical process (patients, nurses, doctors, vendors, etc.) without any further settings. Each would only use the keywords or phrases that they feel most comfortable with. For example, a patient would input symptoms in natural language, while a doctor could query after previous diseases and drug names.
To create this tool, current text analysis know-how needs to be improved and enhanced. It’s not enough to look at keywords such as disease names or symptoms. It’s necessary to understand the relationship between these words. Furthermore, since medical staff uses their own jargon, this should also be included and interconnected with common words.
A suitable algorithm based on text analysis would understand the context, look at modifiers such as negations or conjunctions. These aspects are necessary to avoid false alarms.
The final product of such a tool would be a clearly indexed personal record for each patient. This would include the medical history, together with mentions about family members. Also, it could have a section with the list of past and present medication, which can minimize unwanted interactions.
Machine Learning and Natural Language Processing
As mentioned earlier, medical records contain impressive amounts of data already. Add to that all the data which is not in a structured format—like X-rays, lab results, and more— and the task of scanning through all the information by text search becomes impossible.
The solution is to delegate this to machines and create algorithms which can recognize patterns in a wide array of input medium.
To make diagnoses, doctors make correlations between symptoms and conclude the underlying condition. Diagnoses are based on textbook descriptions derived from studies that are usually a few decades old and generally on a population different from the target.
Now, with the help of machine learning, text analysis services, offered by companies like InData Labs, will mean increased diagnostic accuracy. Furthermore, there is also a chance of discovering new links between previously unrelated conditions.
The Importance of Context
In all text analytics algorithms, context makes the difference. For medical applications, this is even more important as it can define the premises of the analysis. Details like: does the patient have a family history of the disease? Is the patient under treatment with drugs which can cause side-effects? Does the patient have a high-risk job?
All this data is usually noted in their personal records, but not all translate to a medical code. For example, even if there is coding for smoker/non-smoker, the number of cigarettes per day can make a clear difference.
Machine learning goes into details and looks at more than simple keyword triggers. Natural Language Processing (NLP) looks at the whole sentence and tries to understand it, much like Siri or Alexa understand user requirements. The same approach can create a 360-degree view of the patient’s condition by including all documents, medical notes, and history in a single, interconnected repository. Even more, if this repository can be queried in natural language or offer additional filters, this can be a game changer for medical software.
Privacy Issues
The main obstacle against creating a Google-like tool for medical purposes is related to personal data privacy. Current regulations are tight enough to make the creation of such an algorithm difficult. Of course, results can be aggregated and served as an average, but standards rarely serve any purposes in modern medicine, which aims to be personal. Answering these concerns should be a key priority.
Future Developments
When building a text analysis tool for medical records, there are a few goals to keep in mind for the long run. The first is to optimize the platform for search, both in layman’s terms and medical jargon. Next, make it sensible to the context and calibrate the algorithm to recognize modifiers. Last but not least make the tool interoperable as it is less likely to be enhanced just by one team.
Such a system would benefit the patient, on the spot, but would also create a diagnostic database. The records could be used as training datasets for neural networks to teach them to perform diagnosis and prescribe medication based on individual characteristics; a window into a faster, smarter, healthcare system.